Sustainable AI Alignment for Personal and Small-Scale Applications: Leveraging RLHF and Pretraining for Domain-Specific Language Models
This thesis seeks to explore the use of Reinforcement Learning from Human Feedback (RLHF) and pretraining techniques to fine-tune small language models for domain-specific applications. Focusing on data from health, manufacturing, and space sectors, this research will offer insights into how sustainable AI alignment can be achieved for specific uses without the need for large-scale, computationally expensive models.
Contact person
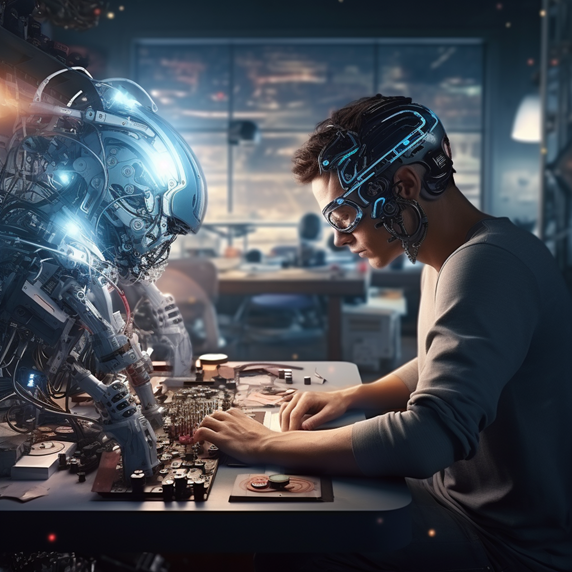
In the current era of large-scale AI models, achieving a balance between model performance and sustainability is a challenge, especially for small-scale or personal use applications. Aligning AI models to perform well on specific tasks without excessive resources is essential.
Research Topic Focus
- Comprehensive study of RLHF and pretraining techniques in aligning AI models.
- Designing methods to fine-tune small language models for domain-specific applications using RLHF and pretraining.
- Evaluating the effectiveness and efficiency of the aligned models in processing and understanding data from health, manufacturing, and space sectors.
- Investigating the sustainability implications of the designed alignment strategies in terms of computational costs, energy consumption, and model robustness.
Expected Results
- A detailed understanding of the potential of RLHF and pretraining in aligning small language models.
- Domain-specific small language models fine-tuned using the proposed techniques that showcase competitive performance.
- Demonstrated sustainability benefits of the alignment techniques in the context of personal and small-scale AI applications.
Learning Outcomes
- Acquire an in-depth understanding of RLHF and its application in AI model alignment.
- Gain hands-on experience in leveraging pretraining techniques for domain-specific fine-tuning.
- Develop expertise in evaluating the sustainability and efficiency of AI models.
- Enhance problem-solving skills in adapting AI models for specific sectors, ensuring both performance and sustainability.
Qualifications
- Strong foundation in AI, with a focus on language models.
- Proficiency in reinforcement learning and pretraining techniques.
- Familiarity with datasets from the health, manufacturing, and space sectors.
- An analytical mindset and dedication to sustainable AI solutions.
References
- Christiano, P., Leike, J., Brown, T., Martic, M., Legg, S., & Amodei, D. (2017). Deep reinforcement learning from human feedback. arXiv preprint arXiv:1706.03741.
- Devlin, J., Chang, M. W., Lee, K., & Toutanova, K. (2018). BERT: Pre-training of deep bidirectional transformers for language understanding. arXiv preprint arXiv:1810.04805.
- Strubell, E., Ganesh, A., & McCallum, A. (2019). Energy and policy considerations for deep learning in NLP. arXiv preprint arXiv:1906.02243.