Due to its vast expanses and rugged terrain, Norway is a country that relies on its extensive network of regional airports for ensuring continued value creation and settlement in its more remote towns and cities. However, the economic viability of smaller airports is being challenged by the modern economic climate, with tower maintenance and inefficient use of air traffic personnel being particularly costly. At the same time, the composition of the air traffic environment is changing, with drones emerging for commercial, civilian and military applications. This means that air traffic controllers (ATCOs) are on the one hand having to deal with increasingly high complexity, while their expertise is not being optimally utilised, due to being physically bound to low-capacity airports. One concept that offers to address these challenges is Remote Tower.
Remote towers, in which air traffic services are provided from a remote location by streaming sensor data from the airport to a remote tower centre, have numerous advantages over traditional towers. They cut costs and increase efficiency by allowing several ATCOs to be housed in a central location, thereby enabling the flexible allocation of aerodromes. They even allow single ATCOs to take responsibility for two or more airports simultaneously. Nevertheless, in order to ensure that safety levels are either preserved or enhanced, it is critical that ATCOs maintain a high level of situational awareness (SA) at all times. In the case of handling multiple airports simultaneously, automation of parts of the ATCOs responsibilities enables them to keep a high level of SA, despite the increased complexity. Such automations, however, require an accurate and real-time digital representation of the airport, covering all aspects of relevance to air traffic control, including traffic, weather and lighting information among other things.
In SESAR PJ.05, SINTEF has focused on creating a unified geometric, visual and semantic representation of the aerodrome that can be used to support SA. This includes seamless integration of the 3D geometry and video streams through precise calibration of cameras, together with cross-camera exposure alignment. Semantic information is added to the digital representation through the use of video tracking of ground and air traffic, based on the latest machine learning models. 3D information is dynamically extracted from the model to support the ATCO’s perception of depth, whilst also linking with the video tracking to provide a complete surface tracking tool that can position detected objects in a map. Other features, including functionality for a fast-forward visual forecast (and fast-rewind recap) of traffic, and visual simulation of predicted lighting and weather conditions were investigated, in order to support dynamic switching of ATCOs between remote airports.
By automating surveillance and helping ATCOs to focus their attention on the most important aspects of a situation, these algorithms can contribute to improving security, capacity, efficiency and flexibility.
Our partners are Saab and LFV.
Read more about the project and all the partners at the main project website here.
Read more about the results here.
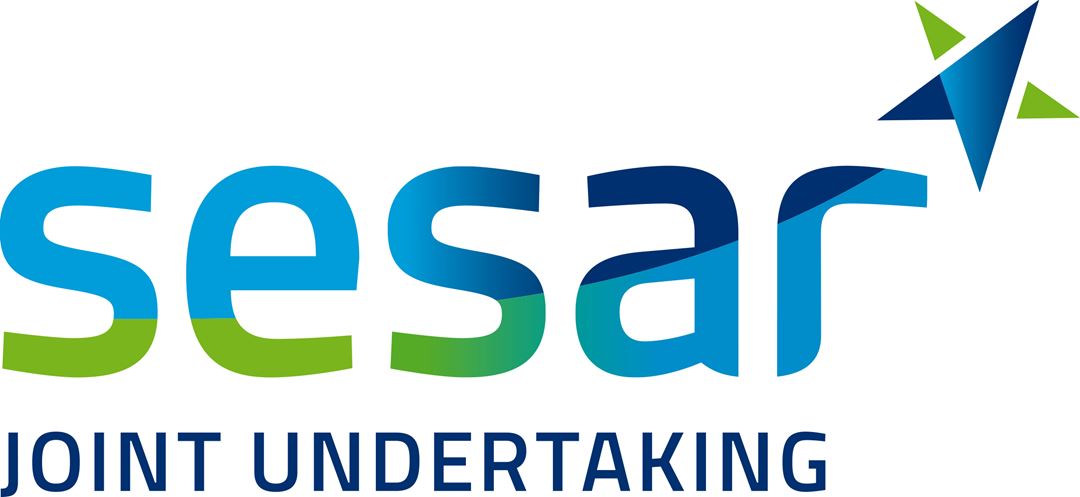