Objective
Develop an adaptive decision support system that improves efficiency of video-based inspections with automatic report generation and extended use of inspection data.
Posicom will use the leading competence of SINTEF and NTNU to extend their existing Seekuence video inspection system with these features:
- ML supported tagging of objects and events, and
- Contextualization of video with additional information.
This will give Posicom a unique advantage in serving the maritime and fish farming market segments represented by DNV, VUVI, Island Offshore and Mainstay.
Background
Companies across oil & gas, maritime and fish farming value chains are seeking quicker, more accessible, and cost-effective ways to ensure technical safety and performance of projects and operations. Increasingly more of those companies are using digital technologies to virtually bring inspectors and surveyors to sites in order to witness and verify the quality and integrity of equipment and assets to company specifications or industry standards.
Partners
SINTEF´s role in the project
SINTEF is responsible for the technical development of early-stage prototypes.
- We are developing a video tagging module, applying Machine Learning (ML) to classify, detect and segment interesting findings (e.g., marine growth) in underwater ship hull inspection videos.
- We are developing a video contextualization module that relates the findings in the inspection videos with additional data in a Knowledge Graph (KG) to support data analytics.
Machine Learning (ML) models
We developed ML models for multi-label image classification, object detection, semantic segmentation, and video classifications.
Multi-label image classification
Develop multi-label image classification ML model to identify relevant findings in ship inspection videos. We support 9 different classes: anode, bilge keel, corrosion, defect, marine growth, overboard valve, pain peel, propeller and sea-chest grating.
Object detection and semantic segmentation
Develop object detection and semantic segmentation ML model to support automatic tagging of objects in ship hull inspection videos (e.g., paint peel as shown in the figure below).
Multi-label video classification
Develop a deep learning ML model that uses spatio-temporal information from videos to improve the automatic video analysis of underwater ship hull inspections. The figure below shows screenshots from a demonstrator that was developed in the project.
Demonstrators
A selection of the demonstrator apps developed in the project are available online.
Video contextualization
- Website: https://liaci-context.sintef.cloud/
- Contextualization demonstrator showing image findings from different ship inspections.
- The vessel names have been anonymized.
Fish farm - hole detection in nets
- Website: https://liaci-fishfarm.sintef.cloud/
- Demonstrator app that detects holes in fish farm net videos
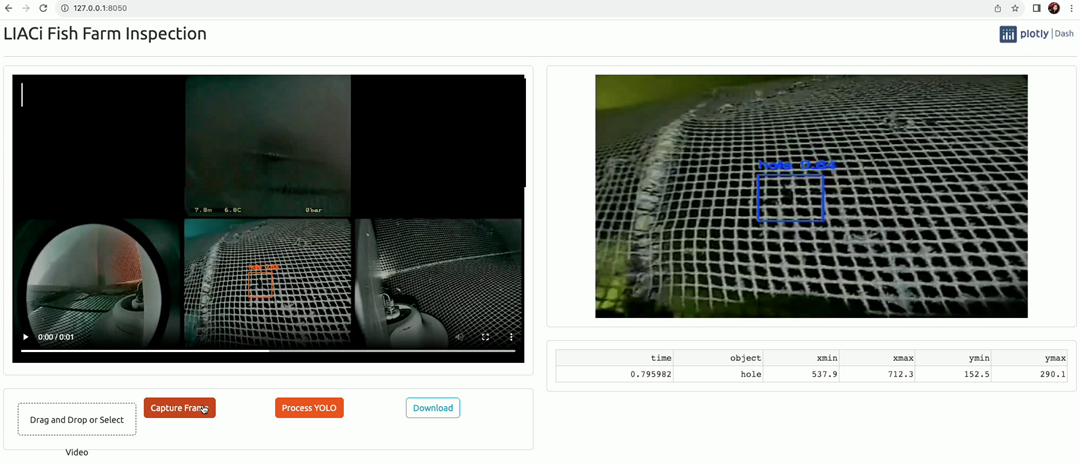
Video quality indicator
- Website: https://liaci-video-quality.sintef.cloud/
- Demonstrator app that shows the video quality based on the Underwater Color Image Quality Evaluation (UCIQE) metric
Datasets
Underwater ship inspections
- Website: https://liaci.sintef.cloud
- First public large-scale semantic segmentation dataset for underwater ship inspections
- Contains 1893 images with pixel annotations
data.sintef.no
3 public LIACi datasets have been published on the data sharing platform http://data.sintef.no
- LIACi dataset for demonstrator apps
- LIACi semantic segmentation dataset for underwater ship inspections
- LIACi underwater ship inspection video snippets
Currently, http://data.sintef.no is accessible to everyone, but registration is required through a Google account. In a future release of the platform, planned during Q1 2024, public datasets will be accessible without requiring Google authentication.
Publications
A list of publications can be found in CRISTIN.
Funding
LIACi has received funding from The Research Council of Norway under the project No 317854.